
Data for all
23 Mar 2021

Dear Reader,
Data is king or so they say. Inclusivity for data platforms can be achieved when data reaches all stakeholders without lag or restrictions. Market information disseminated quickly could make the difference between making and losing money in the financial markets.
Spending on financial market data/analysis and news exceeded the USD 30 billion mark for the first time ever in 2018, according to a new report published by Burton-Taylor International Consulting.
Xignite’s market data cloud is a single platform that unifies financial data consumption. It provides cloud-based financial market data APIs to help emerging companies and established enterprises deliver real-time and reference market data to their digital assets, such as websites and apps. Xignite cloud API’s cover millions of financial instrument across all asset classes.
With all the real time data at hand, investors would like to trade across all asset classes. Robinhood makes it possible to trade in stocks, ETF’s, options and crypto, brokerage free. It also gives access to professional research reports, margin trading and instant deposits. Various other tools and features such as price movement notifications and customized investment news provide additional appeal.
Market Prophit is a financial Big Data analytics company delivering real-time, meaningful intelligence to investors through sophisticated natural language processing, predictive analytics, and powerful visualizations of sentiment and buzz derived from financial related conversations in social media chatter. Market Prophit is the first service ever to provide quantitative rankings of financial bloggers data in social media and generate unique, real-time sentiment signals (bullish/bearish).
Another fintech startup Mindbridge Analytics is using AI and ML to detect anomalous patterns of activities, unintentional errors and intentional financial misstatements in financial datasets. The auditing software will automate ingestion and analysis of data and help accountants identify risk. A risk score is generated for the processed data and will flag transactions that need investigation.
Financial institutions harnessing the power of big data coupled with fintech innovation are leveraging meaningful insights from many disparate data sets. Fintech players have set the ball rolling for financial inclusion. A collaborative approach is the natural next step to accelerate the pace of this process.
Credits : Akhil Handa,Prithwijit Ghosh
Popular Articles
Related Articles

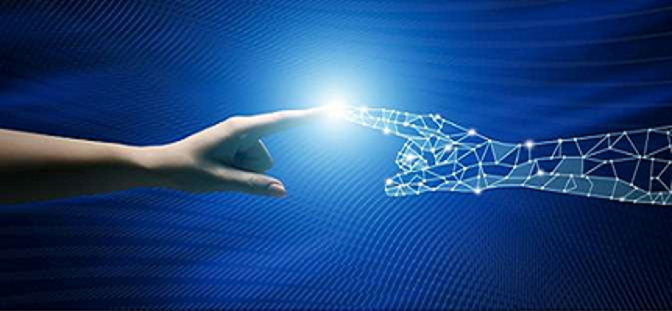


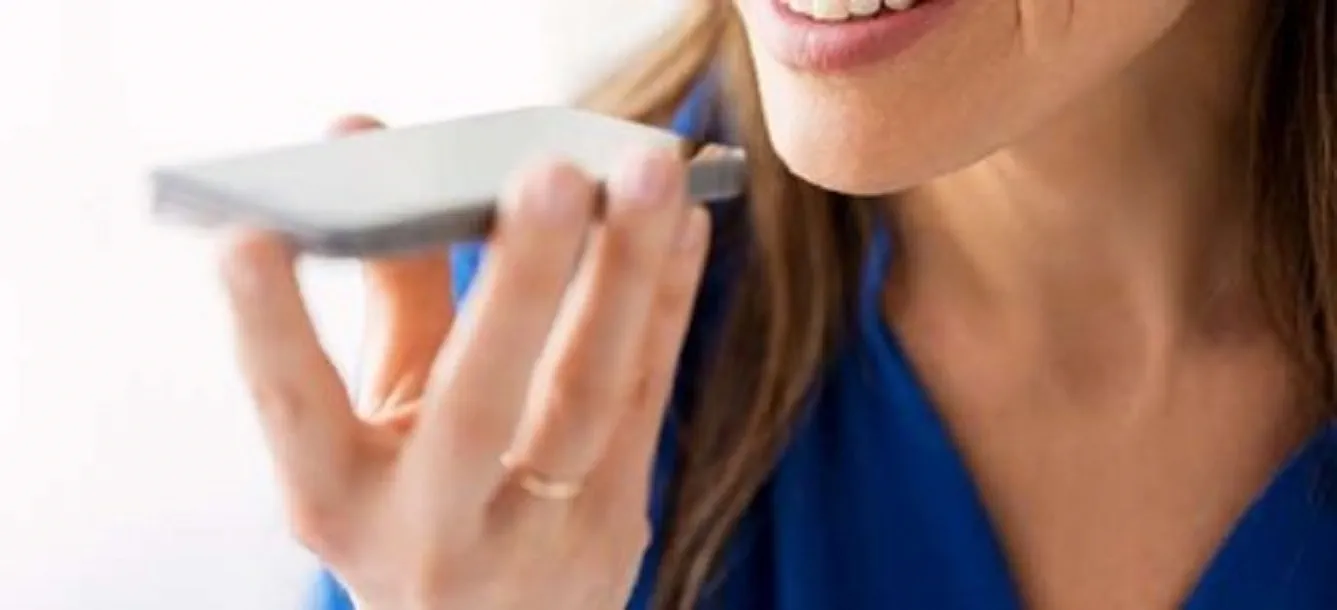


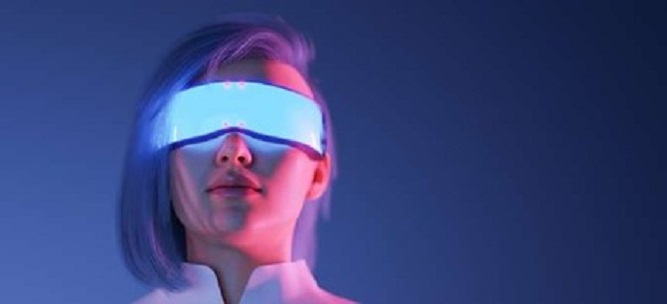


-
Disclaimer
The contents of this article/infographic/picture/video are meant solely for information purposes and do not necessarily reflect the views of Bank of Baroda. The contents are generic in nature and for informational purposes only. It is not a substitute for specific advice in your own circumstances. Bank of Baroda and/ or its Affiliates and its subsidiaries make no representation as to the accuracy; completeness or reliability of any information contained herein or otherwise provided and hereby disclaim any liability with regard to the same. The information is subject to updation, completion, revision, verification and amendment and the same may change materially. The information is not intended for distribution or use by any person in any jurisdiction where such distribution or use would be contrary to law or regulation or would subject Bank of Baroda or its affiliates to any licensing or registration requirements. Bank of Baroda shall not be responsible for any direct/indirect loss or liability incurred by the reader for taking any financial decisions based on the contents and information mentioned. Please consult your financial advisor before making any financial decision.
Contextual Fencing
Dear Reader,
From sending a tailored promotion when a customer enters a retail store to powering order-ahead food delivery, geofencing plays a key role in making mobile apps contextually aware. Geofencing is a location-based service in which an app or other software uses GPS, RFID, Wi-Fi or cellular data to trigger a pre-programmed action when a mobile device or RFID tag enters or exits a virtual boundary set up around a geographical location, known as a geofence.
Think about geo-fencing like an electric fence. In the real-world Geo-fencing allows for the setup of virtual fences or areas, wherein the entry and exit through the areas triggers a response or action by the individual or company monitoring the geo-fence. So, geo-fencing triggers alerts for a business owner when key things happen: a customer visits a store, for example, or the store of a competitor.
BMW is an industry leader in the automobile industry. BMW uses geofencing to enhance their customer service. Cars with BMW Trackstar monitor the position of the vehicle. Once a customer activates their service, the position of their car is tracked and pinpointed every 20 seconds. Customers can use their mobile application to track the location of their car if it’s parked in a garage or somewhere on the city streets. That way they never have to worry about forgetting where they parked. Furthermore, this service also helps prevent theft. Geofencing helps them go the extra mile to provide customer service, which is a profitable strategy.
Apple Maps is constantly tracking our location. Based on our habits, this app can predict where one is most likely to go. For example, if you leave work around the same time every day and drive straight home, Apple Maps will recognize this habit. If the app knows that you’re not home, based on being outside of a geofenced area, it will send a push notification informing how long it will take to get home and what the traffic will be like.
Today’s use of geofencing has evolved beyond simply tracking where a target customer is at any given time. With privacy concerns challenging companies to rethink their use of geofencing, companies like Radar are leading the way in innovating how geofences can improve the user experience.
Having data about consumer behavior can be helpful to design better and personalized products. Uber first implemented the geofencing feature to identify airports to notify people in airports about available cabs and special offers on booking.
In the times to come we expect to see companies use geofencing more strategically to send contextually relevant messages.
Credits : Akhil Handa,Prithwijit Ghosh
Disaster Management
Dear Reader,
We are living in the midst of a revolution. Supervised learning, a branch of Machine learning allows engineers to develop models that can train themselves. In turn, these models are helping solve crisis management problems before disaster strikes.
Technologists have long modeled data to harness machine learning for disaster relief. After the Chernobyl crisis, scientists analyzed satellite imagery and weather data to track the flow of radiation from the reactor. Today’s algorithms far outpace their predecessors in analytic and predictive powers. Machine learning models are able to deliver more granular predictions. NASA has developed the Landslide Hazard Assessment for Situational Awareness (LHASA) Model. Data from the Global Precipitation Measurement (GPM) is fed into LHASA in three-hour intervals. If a landslide-prone area is experiencing heavy rain, LHASA then issues a warning. Analysts then channel that information to the appropriate agencies, providing near-real-time risk assessments.
Roofing material is a major risk factor in resilience to natural disasters. So, a model that can predict it is also one that can predict which buildings are most at risk during an emergency. In Guatemala, models are identifying “soft-story” buildings–those most likely to collapse during an earthquake. “Forecast funding” can mitigate damage by providing the most vulnerable with cash assistance to prepare for disaster. Bangladesh and Nepal are nations that are already implementing this strategy.
Natural disasters, such as earthquakes, hurricanes and floods affect large areas and millions of people, but responding to such disasters is a massive logistical challenge. Crisis responders, including governments, NGOs, and UN organizations, need fast access to comprehensive and accurate assessments in the aftermath of disasters to plan how best to allocate limited resources. To help mitigate the impact of such disasters, Google in partnership with the United Nations World Food Program (WFP) Innovation Accelerator has created "Building Damage Detection in Satellite Imagery Using Convolutional Neural Networks", which details a machine learning (ML) approach to automatically process satellite data to generate building damage assessments. As per Google this work has the potential to drastically reduce the time and effort required for crisis workers to produce damage assessment reports. In turn, this would reduce the turnaround times needed to deliver timely disaster aid to the most severely affected areas, while increasing the overall coverage of such critical services. The World Food Programme was awarded the 2020 Nobel Peace Prize and they thanked Google and its team of engineers in pioneering the development of artificial intelligence to revolutionise humanitarian operations.
The application of machine learning techniques to satellite imagery is revolutionizing disaster relief. Crisis maps and image comparisons are helping relief organizations to deliver aid with precision.
Credits : Akhil Handa Prithwijit Ghosh